“Driving” “Minoritized” Students Out of Stem? How Not to Infer Cause from Correlation
Wooden Stake Point
Wooden Stake Points are my debunkings of one or two key aspects of some published paper: “Wooden Stakes kill vampires. And killing the academic claims and norms that suck the blood out of social science validity and credibility is exactly my goal here.”
Ok, on to the paper:
The Short Version
This paper’s answer to its titular claim is a reasonding “yes!” Don’t go by me, go by one of the authors:
Nonetheless, it is incapable of demonstrating that anything caused anything else, even though it repeatedly reaches causal conclusions. It is a case study in “you can’t infer cause from correlation.”
The Not as Short Version
THEY REALLY WANT THEIR RESULTS TO BE CAUSAL!
This study’s headline claim is causal. Its title asks an implicit causal question. “Do intro courses disproportionately drive minoritized students out of STEM pathways?” (emphasis mine).
Their main analyses examine demographic differences after students receive a D, F, or withdraw before completing (DFW for short) an introductory STEM course (such as calculus or chemistry). Their main findings are: 1. well-established disparities (White men get more STEM degrees than other demographics); 2. everyone is less likely to continue in STEM after receiving a DFW; and 3. “minoritized”1 students (except Asians) are even less likely to continue than are White males who receive a DFW.
I have no reason to doubt the existence of these disparities. The issue is whether any conclusions can be reached about what causes them from the study. Let’s see what the authors say. In the quotes from the paper below, causal language is in the original and purposely emphasized by me:
“we examine nearly 110,000 student records from six large public, research-intensive universities in order to assess whether these introductory courses disproportionately weed out underrepresented minority (URM) students.”
“We provide evidence that these courses may disproportionately drive underrepresented minority students out of STEM, even after controlling for academic preparation in high school and intent to study STEM.”
“A first step in transforming an institution through an equity lens is to identify structures that may inhibit diversity.”
“A next step is to quantitatively analyze whether those structures in fact disproportionately impact marginalized students.”
“Our work asks: within the framework of a large, multiinstitutional database, to what extent do a student’s race/ethnicity, their sex, and their number of Ds, Fs, and/or course withdrawals (hereafter, DFWs) for first term STEM classes impact STEM graduation?”
“it investigates disparate impact of systems based on those categories.”
“but ours is the first study to draw out interactions that indicates a disproportionate negative effect of introductory courses on minoritized students.”
“there is a differential impact of DFWs for these students.”
“we find that DWFs differentially impact students who are women and/or underrepresented minorities. These impacts are negative.”
“female students and URM students are essentially penalized for attributes over which they have no control.”
“Further, the interaction of students’ race/ethnicity and DFW count points towards the presence of troubling institutional effects”
“This approach identifies institutional effects where grades differentially impact students by sex and race/ethnicity.”
THERE IS NOTHING CAUSAL IN THEIR ANALYSES
They had a very large sample, over 100,000 students, which is a strength, but nothing about the study established any causal effect of anything; not the institutions, not the STEM courses, not the “system,”; nada, naked, nothing, nude, in absentia, black hole, vacuum, null, void.
A Brief and (Over)Simplified Foray into the Logic of Causal Inference in Social Science
It is generally possible to reach causal conclusions if one conducts a true experiment, in which people are randomly assigned to different experimentally-created conditions. Random assignment means differences between conditions are unlikely if the experiment is worthless. If differences occur, and the only thing that systematically differs between people is which experimental group they were assigned to, those differences were most likely caused by the differences between the experimental groups. If people perform better on a test after being randomly assigned to a “study a lot” condition than when assigned to a “get drunk with your friends” condition, we can usually safely infer that something about those conditions caused the performance difference.
The Study Was Not an Experiment
It is entirely observational. All results are, therefore, essentially sophisticated correlations (and remember that whole “inferring cause from correlation” thing as you read on). Their main results were reported as logistic regressions (these are basically ordinary regressions adapted for dichotomous dependent variables, which in this case, was whether or not students got a STEM degree). These are high-tech stats that have some advantages over simple correlations, but the core reasons one cannot usually infer cause from correlation still hold.
On Inferring Cause from Correlation Redux
Remember that old adage, “you can’t infer cause from correlation”? Well, its good advice for undergrads, because its mostly true, but it’s not quite completely true. You can. It is just very very very very very very hard (see Julia Rohrer’s excellent Thinking Clearly About Correlations and Causation). A correlation between two variables (say “A” and “B”) can occur for many reasons, only one of which is A causes B. To conclude “A causes B” (in this case, “intro STEM courses cause women and minorities to drop out of majoring in STEM”) you have to rule out all (or at least all plausible) other explanations. That includes having to rule out the existence of confounding variables (unmeasured variables that cause both your predictors and your outcomes).
You need to have, not merely a plausible mechanism,2 but a very strong causal theory with such a mechanism. Here is a case where you have such a strong mechanism that you can infer cause from correlation: You do not need an experiment to know that a correlation between jumping out of an aircraft flying at 10,000 feet without a parachute and death means doing so causes death. We know about gravity and the human inability to withstand crashing into solid ground traveling at around 500mph. We have nothing like this clear mechanism for why students leave STEM (which is probably massively complex and multiply determined).
Rohrer (who may not be some sort of Ultimate Authority but who, imho, does some of the best work on causal inference from statistical models) also recommends: instead of reporting a single model and championing it as “the truth,” researchers should consider multiple potentially plausible alternative sets of assumptions and see how assuming any of these scenarios would affect their conclusions. They did not do this.3 One should minimize the problem of measurement error (a highly technical subject) but: 1. The paper mentions no attempts to do so; 2. a simple manifestation of this is discussed below.
Plausible Alternative Models
There is no onus on me, or anyone, to produce them. The onus is entirely on the research team wishing to make causal inferences to explicitly consider them and attempt to rule them out. Although ruling out all possible alternatives can approach impossible, ruling out some alternative causal models is usually not hard. But they did not do it.
Although it is on the authors, not me, to do this, here are a few alternative explanations they might have considered but did not:
SES, which differs by racial/ethnic groups, may cause both low STEM grades and withdrawals.
Women and students from underrepresented minority backgrounds may enter STEM with different skills and different interests than do White men. These different skills and interests may lead non-STEM options to be more attractive to those who hold them, regardless of experience in an intro course. Women or students from underrepresented minority backgrounds may be more likely than White male students to seek out and find satisfying majors outside of STEM. This could occur in the complete absence of there being any sort of flaw in STEM intro courses. Something very much like this already explains at least part of why fewer high school girls enter STEM in the first place.
Other potential confounders: differences in mental health, other skillsets, other interests, family support/stability. The list of untested confounders is vast and not restricted to the ones I specifically identified.
Worse, although the paper has a long section on “limitations,” none addressed the problems of causal inference from correlational data. The paper has no evidence that the authors even were aware of the problem.
It Goes Downhill from There
Seven of the 8 tests across two models of whether DWF’s differentially affected White males versus other students were nonsignificant. The 8th had a p-value of .033; in Psychology, p-values above .01 do not have a great replication record. In fairness, they performed tests of statistical significance designed to protect against false discoveries. However, read on for more information that might influence your judgment about how seriously to take this.
The study was not pre-registered. Although pre-registration is not a silver bullet, in the absence of a publicly-available pre-registered methods and analysis plan, readers cannot know how much flexibility (also known as “p-hacking” or “garden of forking paths”) the researchers exercised while conducting their analysis. We cannot know whether the reported analyses constitute the only ones performed or are selective in some way. Such undisclosed flexibility has an ugly history in social science.
They also seem to have purposely created measurement error when they did not need to. Without getting all technical, measurement error basically means some variable is not a pure measure of whatever the researchers are trying to measure. When two thermometers in the same place give different temperatures, you have measurement error in at least one. This problem is far more severe in the social sciences, which use fuzzy and imperfect measures all the time. In this study, they lumped all students into two categories: 1.received a DFW or 2. completed the courese with some other grade. That lumps in D’s, F’s & W’s into a single category when they are not the same; it lumps As, Bs, and Cs together into another single category when they are not the same.
Here is Rohrer on the effects of measurement error:
“Measurement error can affect all methods of statistical control.”
“…the false positive rate for an effect [i.e., finding a statistically significant effect when the real relationship is zero] can reach very high levels, approaching almost 100%.”
“…the false positive rate increases when sample sizes are large.”
A very specific way this (shall I say it?) “intersects” with the blindness produced by social justice dogmas is discussed below.
Its Peer Reviewed!
It would be useful to start by trying to figure out why students from certain groups differ in their interest and willingness to pursue STEM courses. The authors make this bizarre claim in their discussion:
“In an equitable education system, students with comparable high school preparation, intent to study STEM, and who get Cs or better in all their introductory STEM courses ought to have similar probabilities of attaining a STEM degree.”
Its bizarre on so many levels, one of the most obvious being that people from different groups often have different backgrounds, experiences, and cultures and this can lead them to be interested in different things. This is so obvious that, when it serves social justicey-interests, it is readily deployed in calls for things like positionality statements. So, no, Virginia, a perfectly fair system would not necessarily produce identical outcomes by group.
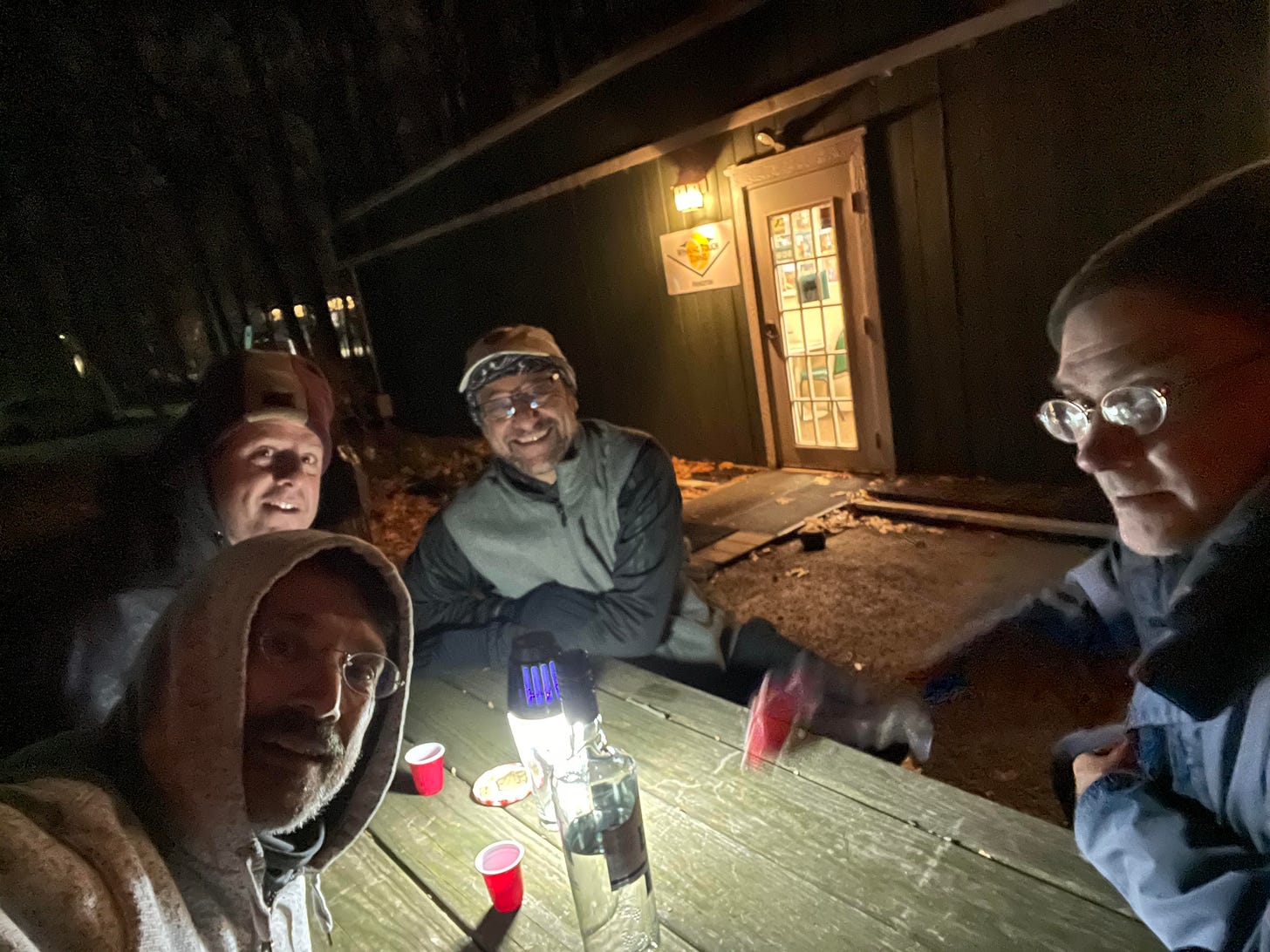
I now return to the measurement error issue. Dichotomizing students into: 1. DFW; 2. ABC builds in measurement error because D is not the same as F or W and A is not the same as B or C. Although I am sure they did not sit around thinking, “How can we add measurement error to our predictor?” they nonetheless intentionally created a variable with measurement error unnecessarily.
I’d bet dollars to donuts that: 1. Students with B’s are more likely to continue than those with C’s and those with A’s are more likely to continue than those with B’s; and 2. There are some demographic differences in grades, even if the sample is restricted to C or better. And W (withdraw) is an entirely different animal than sticking out a course and getting a grade. People do withdraw rather than get a low grade, but they also withdraw because, when faced with the actual material, sometimes, they are like, “This is not what I thought it would be like or want to be spending my time on!” Couldn’t there be demographic differences in interests?
Of course, maybe there is some injustice going on somewhere. Injustice can produce unequal group outcomes. It would just be nice if some evidence of injustice was actually produced, rather than relentlessly overinterpreting statistically souped up correlational evidence of longstanding disparities as causal effects of intro courses “weeding out” minorities in STEM.
Remember, this paper was published The Proceedings of the National Academy of Science, one of the most prestigious and influential outlets in all of academic peer reviewed publishing. The failure to acknowledge the correlation-causality problem, or to require the authors to walk back their cascade of unjustified causal claims, is an epic failure of peer review.4
Bottom Lines
The disparities the study reported are credible mainly because they are consistent with lots of prior research showing their existence.
It is reasonable to ask (scientifically, socially, and politically) about the extent to which such disparities reflect ongoing discrimination or unfair obstacles, and, if so, in what part of the education process.
The paper was duly published and the data are publicly available and no one has identified any fraud or errors. Its conclusions may be entirely or partly wrong or unjustified, but it should not be retracted.
All of its causal claims might be true.
The published paper provides no scientific basis for believing that any of its causal claims actually are true.5
“Minoritized” is social justice-speak for what everyone else would call “minority.” The idea is that … well, I am not sure what the idea is, because its logic breaks down quickly. It is meant to convey that being a minority is imposed by society, not something about the person. But try asking Americans who are Black, or have other ethnic minority heritage (Chinese, Korean, Mexican, Cuban, Jewish, etc.) if that has been “imposed” on them, and get back to me with their response. The use of the term here (and elsewhere) is a tell that what is going on is less a concern for scientific truths than for justifying political activism.
Academics are very good at making almost anything, no matter how ridiculous, seem plausible, so mere plausibility is a nearly useless standard. They are not alone in this ability.
Alternative models. They ran no models testing alternative causal processes. They did run models with three versus five levels for their race/ethnicity variable.
The paper does include this statement at the beginning of a section titled “Future Work”: “More research is needed to establish a causal link and explore interventions that may level the playing field.” Ya think?
From My Positionality Statement
My default positions are:
Not to believe anything published in social science without compelling reasons.
This goes double for anything that affirms leftwing worldviews, not because the left is any more biased than the right, but because the leftwing monoculture in most of the social sciences has short-circuited skeptical vetting writ large.
It sounds like an interesting dataset. I would be interested in assessing completion stats by category (sex, race, nationality, ethnicity, ses, parental religiosity, and parental political views) vs persistence in STEM as a function of grades. It would also be interesting to see how the comparative strength in verbal aptitude correlated. My prediction is that the better the relative score on the verbal portion of the SAT is to the math score the less likely that a marginal student will persist in STEM. I also predict that the more need dependent aid a student receives, the less likely the student will persist as a function of grade (at my institution students have to retain a 3.0 gpa to keep institutional and state financial aid - students with low grades in intro calc, chemistry, and physics disproportionately leave for easier majors the more dependent they are on aid to stay in college).
I suspect religion and politics play a role as well. Ecklund’s work showed that the childhood religiosity predicts adult religious practice just as well for elite scientists as the general population. Thus the underrepresentation of religious scientists is likely a selection effect (I bet a study on political views would show something similar). Thus one might expect that a student who from a religious home would be more on the fence for STEM. Poor intro grades would thus have a disproportionate effect as it relates to persistence (ie, they are more likely to hop to business when the going gets tough). Since black and hispanic americans are more religiously observant than their white counterparts (who are more observant than their Asian counterparts), I predict that this will contribute to persistence as a function of race/ethnicity.
So I can think of three factors that could impact persistence in STEM that correlate with race among students who earn a DFW in intro courses: parental SES, religiosity, and comparative verbal skills. Maybe systemic racism drives some or all of these, but even if this is the case, that isn’t related to what happens in the intro courses.
Thanks Lee. Unfortunately, for.those who who lack a background to understand the methodological failures, the fact it's in a fancy journal and has lots of cool sounding terms, not to mention a conclusion that they already belive, means it will spread far and wide while those asking questions will be ignored. Your work is vital, especially because you are not particularly ideological. Please.keep up the.good.fight